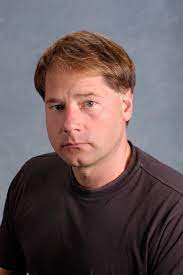
Bart Kosko
Bart Andrew Kosko is a writer and professor of electrical engineering and law at the University of Southern California (USC). He is a researcher and popularizer of fuzzy logic, neural networks, and noise, and author of several trade books and textbooks on these and related subjects of machine intelligence. Kosko holds bachelor's degrees in philosophy and in economics from USC (1982), a master's degree in applied mathematics from UC San Diego (1983), a PhD in electrical engineering from UC Irvine (1987) under Allen Stubberud and a J.D. from Concord Law School.
He is an attorney licensed in California and federal court, and worked part-time as a law clerk for the Los Angeles District Attorney's Office. Kosko is a political and religious skeptic. He is a contributing editor of the libertarian periodical Liberty, where he has published essays on "Palestinian vouchers".
Kosko's most popular book to date was the international best-seller Fuzzy Thinking, about man and machines thinking in shades of gray, and his most recent book was Noise. He has also published short fiction and the cyber-thriller novel Nanotime, about a possible World War III that takes place in two days of the year 2030. The novel's title coins the term "nanotime" to describe the time speed-up that occurs when fast computer chips, rather than slow brains, house minds.
Kosko has a minimalist prose style, not even using commas in his book Noise. Kosko's technical contributions have been in three main areas: fuzzy logic, neural networks, and noise.
In fuzzy logic, he introduced fuzzy cognitive maps, fuzzy subsethood, additive fuzzy systems, fuzzy approximation theorems, optimal fuzzy rules, fuzzy associative memories, various neural-based adaptive fuzzy systems, ratio measures of fuzziness, the shape of fuzzy sets, the conditional variance of fuzzy systems, and the geometric view of (finite) fuzzy sets as points in hypercubes and its relationship to the ongoing debate of fuzziness versus probability.
In neural networks, Kosko introduced the unsupervised technique of differential Hebbian learning, sometimes called the "differential synapse," and most famously the BAM or bidirectional associative memory family of feedback neural architectures, with corresponding global stability theorems.
In noise, Kosko introduced the concept of adaptive stochastic resonance, using neural-like learning algorithms to find the optimal level of noise to add to many nonlinear systems to improve their performance. He proved many versions of the so-called "forbidden interval theorem," which guarantees that noise will benefit a system if the average level of noise does not fall in an interval of values. He also showed that noise can speed up the convergence of Markov chains to equilibrium.
Best author’s book
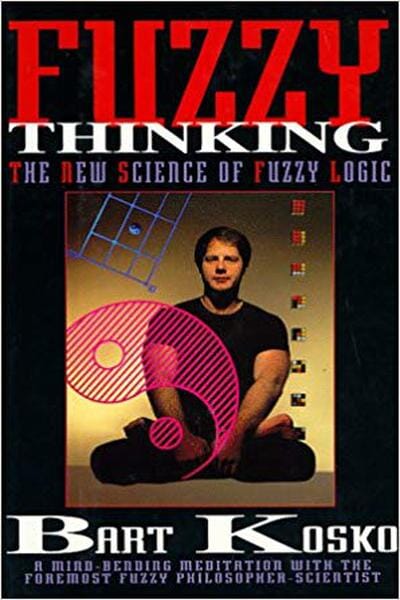

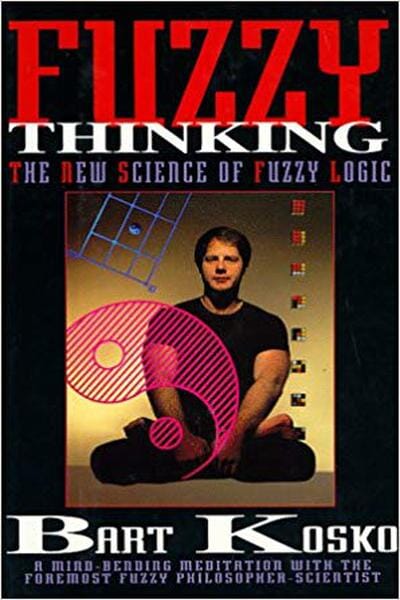